Classifying Aluminum Scrap Metal Using Magnetic Induction Spectroscopy
- Posted by
Red Pitaya Team
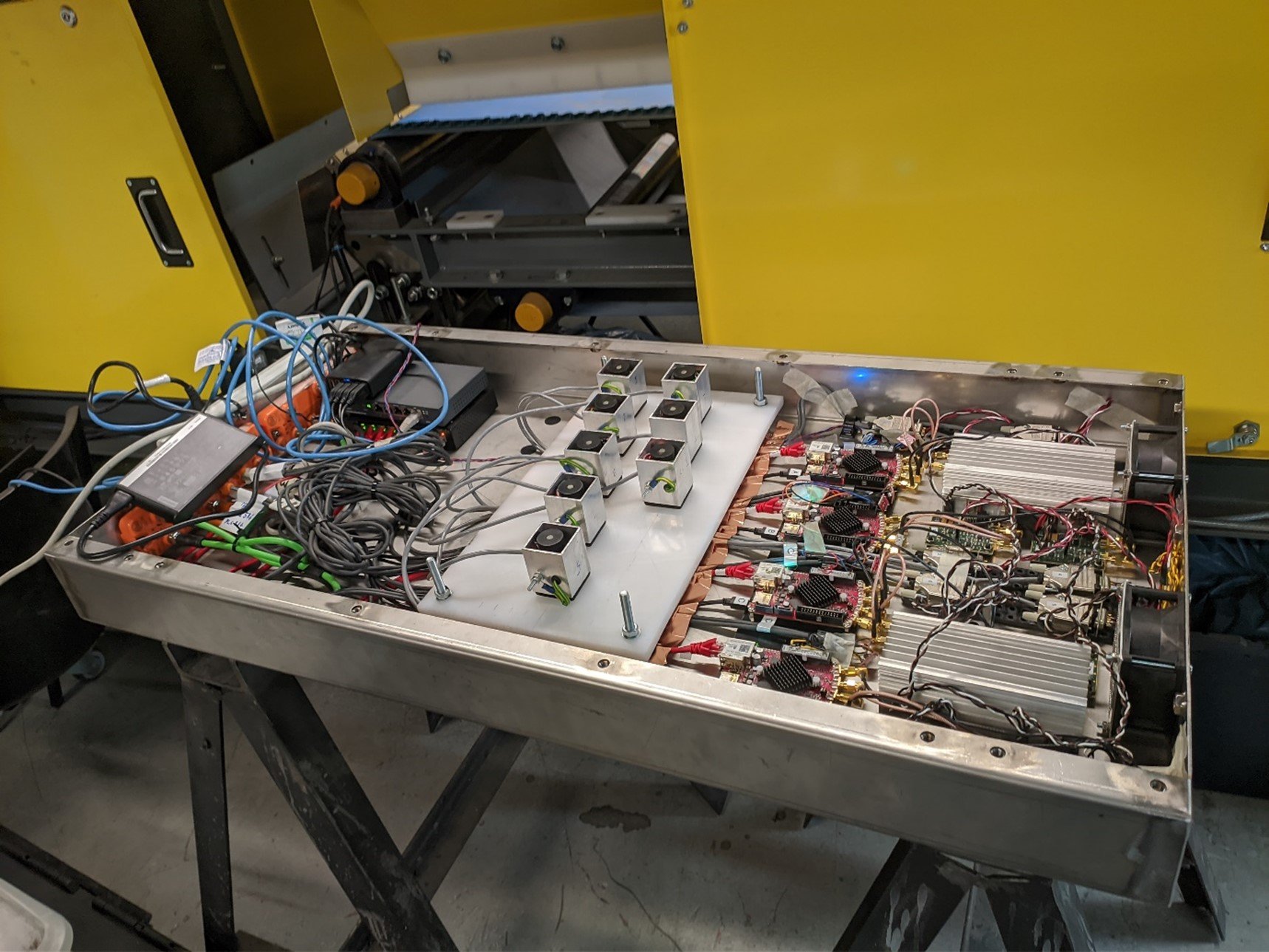
Tropical summers, torrential rainfall, floods… Climate change is becoming more and more visible, and concepts such as the circular economy and waste recycling are no longer strangers to our daily vocabulary. Not only is our awareness of the problem growing, but the technologies used for waste recycling are also becoming more efficient, with a little help from Red Pitaya.
Aluminum is one of the key materials when it comes to reusing obsolete products, rather than producing new ones. But while the process for shredding and recovering virgin metal is relatively easy, the original process used to manufacture the aluminum parts also plays an important role here. Wrought and cast aluminum are the two main material categories, and both types must be separated during recycling to prevent the impurities present in cast aluminum from contaminating the wrought product. This contamination can lead to a significant degradation of the material properties, making it less useful for important applications, such as in the automotive industry, where the demand for wrought aluminum is on the rise.
Several methods are currently in use to separate the two categories in a stream of shredded aluminum: manual sorting is a rather slow process, with its efficiency mainly depending on the skill of the worker, while some technological tools – such as LIBS (Laser-Induced Breakdown Spectroscopy) and XRF (X-Ray Fluorescence) – allow performing the task at an increased pace, but with an accuracy that is sensitive to surface contamination.
More cost-efficient solutions use the difference in conductivity – expressed as a percentage of the conductivity of copper, called ICAS – between cast (23% ICAS) and wrought (40% ICAS) aluminum to differentiate them. This process is reported by K.C. Williams et al. in the paper Classification of Shredded Aluminium Scrap Metal Using Magnetic Induction Spectroscopy.
The article explains how a straightforward distinction between the two types of aluminum can be achieved, applying a uniform oscillating magnetic field on a conductive sphere in free space to induce eddy currents, and measuring the resulting secondary magnetic field. An example can be found in the graphs in Figure 1, showing real and imaginary components for cast and wrought aluminum spheres.
Figure 1: Real and imaginary components of secondary magnetic field, produced by cast and wrought aluminum spheres
To go from a well-defined volume to a stream of randomly shaped and sized parts, an experimental setup was designed and assembled to apply multi-frequency magnetic induction spectroscopy for aluminum sorting. After an initial test on static objects, the setup was integrated in an industrial conveyor system, where aluminum parts with different origins were traveling at a speed of 2 m/s. The concept is shown in Figure 2 and basically consists of a detection system – called MetalID – for material differentiation and air ejectors at the end of the conveyor belt, making sure each type ends up in the right bin.
Figure 2: Diagram of the separation system, with the MetalID setup integrated
The components required for this experiment can be seen in Figure 3 below, with eight measurement coils and four STEMlab125-14 units clearly standing out. The coils consist of concentric excitation and reception windings that simultaneously generate the primary field and pick up the secondary field. The Red Pitaya units are in charge of producing the multi-frequency primary signal via their DAC and analog outputs, while the secondary voltage is connected to their analog inputs and subsequent ADC for FFT, post-processing, and material classification. An industrial PLC then allows the STEMlabs to trigger the air ejectors. During an initial training period, measurements were first downloaded from the Red Pitaya units onto a PC, after which different machine-learning models, developed by the same computer, were implemented back onto the STEMlabs to allow autonomous operation for material classification.
Figure 3: The MetalID entrails, including eight ID coils and four STEMlab125-14 units, among others
The results of the different test runs show that the static system achieves satisfactory performance, with recovery and purity rates (representative of the accuracy) of 89.66% and 94.96%, respectively. However, these results could not be repeated on the moving conveyor system, where the test samples produced a recovery rate of 63.89% and purity rate of 60%. An assessment of the results identified three main areas for improvement. The first two areas are linked to physical issues, as they are the position of the sensor with respect to the metal piece, a factor that induces false results, and the influence of the orientation of the part on the measurement. The third area for improvement is related to changing the algorithm used in the machine learning process. As always, innovative processes in fields requiring fast development curves are subject to trial and error. Fortunately, it seems the authors already know where to look to make future experiments more successful, with no weaknesses found in the STEMlabs’ performance.
Article: Classification of Shredded Aluminium Scrap Metal Using Magnetic Induction Spectroscopy (K.C. Williams et al., The University of Manchester, U.K.)